Introduction
Making decisions that are supported by evidence and data is usually a good thing, one that must be encouraged. It is often objective, clearly puts different stakeholders on the same page quickly to facilitate buy in and action, takes out individual biases and group think out of the equation…leading to better decisions, most of the time. However, what if the data itself is right, but what it says is wrong?
Survivorship Bias: Lessons from the World War 2
Imagine it is World War 2 and the US is losing a lot of fighter planes under attack. You are asked to identify the weakest part on the body of the plane which must be reinforced to withstand hits, saving planes and people. You take a look at all the planes that came back “wounded” and noticed that on almost all these planes, the underside of the left wing was badly damaged. You reason that, based on data, this part of the plane was more likely to be attacked and badly damaged leading to loss of planes and lives. Therefore, based on data, the US needs to reinforce the underside of the plane’s left wing.
Great? Not so fast! When the above scene unfolded in real life, Mr Abraham Wald challenged the decision after making the following two key observations:
- the sample was biased as it was made up of only the planes that “survived” the battle field.
- if these planes had survived, then the damaged areas could not be the critical ones.
He inferred and concluded it was more likely that the critical parts of the plane requiring additional reinforcement were those that were not damaged on the “surviving” planes. By ignoring the “non survivors”, this crucial piece of information was forgotten. The sample only considered survivors and painted a distorted reality, leading to a wrong conclusion/decision.
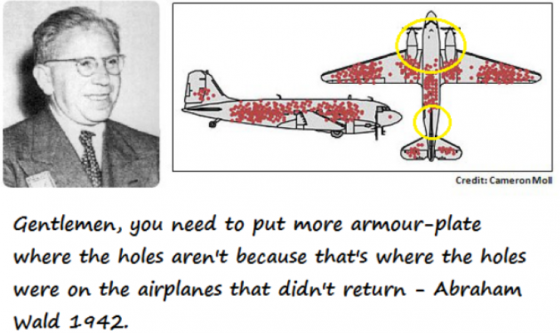
Ladies and gentlemen, this tendency to focus on survivors and ignore non-survivors is called survivorship bias.
Survivorship Bias in Action: The case of Social Media Data
The World War 2 is over, but the survivorship bias still persist. Today, advanced telecommunications technology, improved smartphone penetration and popularity of apps has elevated the role of data in our lives. This has created a new “playground for both young and old”. Social media demands our attention. Facebook, Twitter, LinkedIn and Instagram has over 2billion, 300million, 600million and 1billion active users worldwide respectively. These numbers are significant and drive the generation of big data which, with the help of smart tools, can help us make better decisions (sometimes). The market for social media analytics is now close to US$5bn and is expected to grow at compounded annual growth rate (CAGR) of almost 30% over the next 5years. Yet, depending on where you live and what you are looking for, social media insights remain susceptible to risks of survivorship bias.
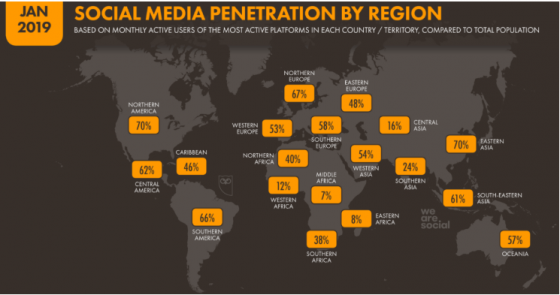
The distribution of social media users is not uniform and as a consequence, the value of the resulting insights is not uniform. The social media penetration in North America and East Asia is about 70%, but ranges from 7% to 40% for Africa (picture). A 7% social media penetration might represent a form of “survivorship bias” whereupon only the views of an elite few are amplified, chopped and diced to draw insights that are hardly meaningful nor representative…forgetting everyone else. This 7% might be in a totally different class than the other 93%, with different behaviours, affordability, taste, preferences, experiences and aspirations. Although the input data is correct, the resulting insights can lead to wrong and costly business and civic decisions.
Conclusion
Data driven decision making is a great concept, but is not fool proof. The data can be right, but what it says can be wrong because of survivorship bias. Wrong decisions should be avoided, they are costly for business, the environment and society. Those who are privileged enough to lead and their advisors, must exercise responsibility when handling and interpreting data. Survivorship Bias is one of the many pitfalls they should keep an eye on.
They must:
- be aware of the sources and risks it poses.
- resist the blind seduction of analytic tools and always consider context (geographic, demographic, client/customer, product/service etc).
- maintain a dose of healthy skepticism. Insights from tools have to make sense; triangulation and sense checking should be allowed to validate outputs.
by Grant Chivandire
Comments
2 responses
You learn something new everyday… Thank you
Data never “says” anything. It’s interpretation that is the source of bias. That’s why 5 people can interpret the same report/dataset and have 5 completely different deductions.